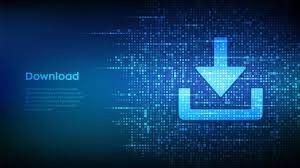
Additionally, we perform experiments on aligned noise distributions and the generalization of the designs to unseen noise distributions. “In-network” combines both tasks at feature level, while “pre-network” first performs denoising and then super-resolution. The goal of this project is the investigation of two architectural designs to jointly perform denoising and super-resolution. Deep Learning Architectural Designs for Super-Resolution of Noisy Images : In my Master Thesis, I developed the Quantile Sparse Image (QuaSI) prior and its adaptive version (AQuaSI) under the supervision of Thomas Köhler. To improve deep learning architectures, especially the extension of a recognition network with additional tasks from the field of image processing shows great potential. This is specifically useful in real-world scenarios, where the image acquisition is unknown. I started to focus on robust deep learning methods that can reliably predict test data that slightly deviates from the training data. Participating in the International Computer Vision Summer School (ICVSS) 2018 sparked my interest in deep learning.
ADMM LAB MASTER LICENSE
Within the last three years, I worked on the super-resolution of indecipherable license plates and license plate recognition. Recent Projects:īelow you will find my recent projects. PhD candidate in the multimedia security group and member of the SFB/TRR 89 Invasive Computing Project C5 “Security in Invasive Computing Systems“.
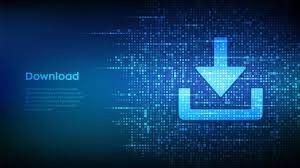